The Evolution of Artificial Intelligence: From Turing to Deep Learning
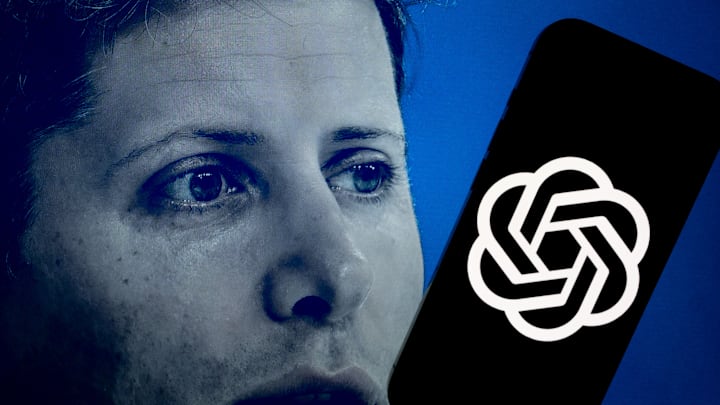
Artificial Intelligence (AI) has evolved significantly since its inception, driven by advances in computing power, algorithms, and data availability. The field traces its roots to the 1950s when Alan Turing proposed the Turing Test as a measure of machine intelligence. Early AI systems relied on symbolic reasoning and rule-based approaches to mimic human cognition. However, progress was limited by computational constraints and the complexity of real-world problems.
The advent of machine learning in the 1980s marked a turning point in AI research. Machine learning algorithms enabled computers to learn from data and improve their performance over time without explicit programming. Early successes in tasks such as handwriting recognition and speech understanding demonstrated the potential of machine learning to tackle complex problems.
In recent years, deep learning has emerged as a dominant paradigm within AI. Deep neural networks, inspired by the structure of the human brain, are capable of learning intricate patterns and representations from vast amounts of data. Breakthroughs in image and speech recognition, natural language processing, and game playing (e.g., AlphaGo) have showcased the power of deep learning in achieving human-level performance in specific domains.
The rise of big data and advancements in hardware, such as GPUs (Graphics Processing Units), have accelerated the progress of AI. Companies and research institutions are leveraging AI for applications ranging from autonomous vehicles and healthcare diagnostics to personalized recommendations and financial trading. Ethical considerations, including bias in AI algorithms and the impact on jobs, continue to be debated as AI technologies become more pervasive in society.
Looking forward, AI research is exploring areas such as explainable AI, reinforcement learning, and AI ethics. Explainable AI aims to make machine learning models more transparent and understandable to humans, enhancing trust and accountability. Reinforcement learning focuses on teaching agents to make decisions through trial and error, leading to advancements in robotics and autonomous systems. Addressing ethical challenges will be crucial in ensuring that AI benefits humanity while mitigating potential risks.
In conclusion, the evolution of AI from early symbolic systems to deep learning represents a journey of innovation and discovery. As AI continues to advance, its impact on society, economy, and culture will be profound. Balancing technological progress with ethical considerations will be essential as we navigate the future of artificial intelligence.